Insight: the journey ahead for AI
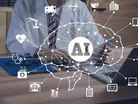
AI technology is receiving increasing attention worldwide and not least in China, stimulating heavy investments from government and industry alike. On the other hand, however, some people think that the scientific essence of AI needs to be upgraded, otherwise, its development will be limited.
In the below contribution, Professor Jinghai Li discusses the potential of this development and where it might go next. Li is President of National Natural Science foundation of China, a state funded agency for fundamental research.
Li has recently been made a judge for the prestigious Queen Elizabeth Prize for Engineering – a £1mn prize which rewards and celebrates the engineers responsible for a ground-breaking innovation in engineering that has been of global benefit to humanity.
The Artificial Intelligence boom
AI (Artificial Intelligence) has developed rapidly in recent years, but it is not a new notion. The concept of AI can be traced back to near 60 years ago, though today’s AI is not as it used to be. The more recent success of AI is due to the boom of big data and the advancement of supercomputing, rather than a fundamental science breakthrough. The basic principle of AI is to learn a model from big data in an inductive way, and then apply the learned model to make prediction on unseen data. This is essentially a statistical modelling methodology, i.e. designing a correlative formula by fitting a model to empirical data.
Deep learning (DL), as a mainstream in AI technique, applies Artificial Neural Networks (ANN) to train a complex model based on massive datasets, which is still a statistical modelling approach in essence. The working principle of DL is to optimize the weights of ‘hidden layers’ connecting inputs and outputs, and the prediction accuracy could be growingly improved by increasing hidden layers. In general, the deeper layer structure and larger training dataset we employ, the higher prediction accuracy DL will achieve. In fact, increasing number of layers is to increase the resolution of analysis equivalent to reducing grid size in computational fluid dynamics, without introducing any intrinsic physical mechanism. It is worth noting that the complex multi-layer network structure used by DL requires more computational resource. Therefore, the development of large-scale GPU parallel computing promotes the progress of AI in some sense.
See also:
Samsung launches new AI fund, NEXT Q
SenseTime and Alibaba to build AI Lab in Hong Kong
Chinese AI startup SenseTime valued at $3bn
The journey ahead
Despite the success, existing AI techniques have two shortcomings. First, the prediction accuracy of AI depends on the size and coverage of training dataset, and it cannot make reasonable prediction on the patterns beyond training dataset. Second, the generality of AI is very limited, i.e., a specific AI technique devised for one field can hardly work well in other fields in general. The science policy makers and business leaders should be aware of these two limitations, and invest fundamental studies in intrinsic physical models based on the logic behind the datasets.
ANN is a hypothetical structure of interconnecting neurons, and we do not know whether it is suitable to the logic and structures of practical problems or not. It is lacking of physical principle behind the complexity of problems, and thus the development of such methodology will be limited in the future.
Mesoscience, proposed in recent years, holds a viewpoint that the complexity of our world is the result of the compromise in competition between different dominant mechanisms in complex systems. This principle is believed to be also suitable for cognitive science. Besides, mesoscience believes that the complexity of our world exhibits a multilevel characteristic and each level has multiscale structure. The complexity is always appearing at the mesoscale in between the unit scale and the system scale at the specified level, which is physically governed by the principle of coordination in competition.
In view of above discussion, by introducing the concept of mesoscience or something equivalent into AI, it is hopeful to provide a scientific foundation for AI, aiming to reflect the logic and structure of the data’s complexity. Concretely, we can determine the number of ANN layers through extensive data analysis at first, that is identifying physical levels, and then conduct a multi-scale analysis on each layer’s structure based on the principle of compromise in competition. We believe this will be one of the possible directions of AI development in the future.